This section allows you to view all posts made by this member. Note that you can only see posts made in areas you currently have access to.
Messages - Flee
Pages: 1 ... 353637 3839 ... 520
1081
« on: January 15, 2018, 07:28:05 AM »
Honestly, as long as your not using magic I have no issue with how you play.
do utility spells/pyromancies count
power within, cast light, aural decoy, etc.
Nah. People just tend to dislike the offensive magic builds because they make the game easy mode. Not only do you do massive damage pretty quickly, but you also don't really need to mind many of the fundamental / challenging aspects of the game. Using spells does not consume stamina so you don't have to worry about managing that while attacking and blocking/dodging. Catalysts and talismans cannot be upgraded so that eliminates the need of getting titanite, making decisions about the upgrade path you're going to take or spending souls on improving your weaponry (which frees up a lot of resources that can go to leveling up or improving your shield/armor instead). There's only attack for each of them (R1) which completely eliminates learning new playstyles, trying out new weapons with different movesets to find out what works and doesn't work for you, and having to use different attacks on different enemies or in response to different moves by bosses. Spells are solid and effective against all bosses, even those with higher resistance to it like Seath and Butterly. Just about every enemy (and especially boss) in the game is most dangerous at close distance, making it so that staying back and slinging some spells their way is a very easy of beating them without really having to learn their moveset, anticipate and react accordingly (and fast), and manage your stamina and timing while up close to them.
Some of the stronger spells in the game (crystal spear, crystal soulmass, pursuers and dark bead) can easily do between 1000 - 2000 damage. Know that Gwyn, the final boss of the game, only has a little over 4000 hitpoints and you might get an idea of how how quickly and easily you can breeze through many of the fights in the game. And yeah, It's true that melee weapons can also do enormous damage. But they're slower, put you in more danger right next to the enemy, require much more specific (glass cannon) builds and are much more difficult to play since they'll essentially require you to be naked at sub 25% health with power within ticking down the seconds and whatnot.
Everyone should obviously play the way they want, but that doesn't change that there are certain playstyles that make the game a lot easier because they allow you to ignore several of its aspects and don't require you to come to grips with many of the more challenging and intricate mechanics. So when you can dish out more damage than the heaviest great axe from relative safety at the other side of the room without ever having to worry about weapon upgrades, stamina management or even learning how to dodge and find openings in the opponent's moveset most of the time, then it's easy to see why many consider it easy mode and feels like it kind of cheapens the experience (especially when on your first playthrough).
B+
Fuck that. A- at least.
1082
« on: January 15, 2018, 05:57:58 AM »
Honestly, as long as your not using magic I have no issue with how you play.
do utility spells/pyromancies count
power within, cast light, aural decoy, etc.
Nah. People just tend to dislike the offensive magic builds because they make the game easy mode. Not only do you do massive damage pretty quickly, but you also don't really need to mind many of the fundamental / challenging aspects of the game. Using spells does not consume stamina so you don't have to worry about managing that while attacking and blocking/dodging. Catalysts and talismans cannot be upgraded so that eliminates the need of getting titanite, making decisions about the upgrade path you're going to take or spending souls on improving your weaponry (which frees up a lot of resources that can go to leveling up or improving your shield/armor instead). There's only attack for each of them (R1) which completely eliminates learning new playstyles, trying out new weapons with different movesets to find out what works and doesn't work for you, and having to use different attacks on different enemies or in response to different moves by bosses. Spells are solid and effective against all bosses, even those with higher resistance to it like Seath and Butterly. Just about every enemy (and especially boss) in the game is most dangerous at close distance, making it so that staying back and slinging some spells their way is a very easy of beating them without really having to learn their moveset, anticipate and react accordingly (and fast), and manage your stamina and timing while up close to them. Some of the stronger spells in the game (crystal spear, crystal soulmass, pursuers and dark bead) can easily do between 1000 - 2000 damage. Know that Gwyn, the final boss of the game, only has a little over 4000 hitpoints and you might get an idea of how how quickly and easily you can breeze through many of the fights in the game. And yeah, It's true that melee weapons can also do enormous damage. But they're slower, put you in more danger right next to the enemy, require much more specific (glass cannon) builds and are much more difficult to play since they'll essentially require you to be naked at sub 25% health with power within ticking down the seconds and whatnot. Everyone should obviously play the way they want, but that doesn't change that there are certain playstyles that make the game a lot easier because they allow you to ignore several of its aspects and don't require you to come to grips with many of the more challenging and intricate mechanics. So when you can dish out more damage than the heaviest great axe from relative safety at the other side of the room without ever having to worry about weapon upgrades, stamina management or even learning how to dodge and find openings in the opponent's moveset most of the time, then it's easy to see why many consider it easy mode and feels like it kind of cheapens the experience (especially when on your first playthrough).
1083
« on: January 14, 2018, 06:37:55 PM »
Fuck the quadpost / monologue, but I'm going to take some of the stuff I wrote here and reuse it for an article I'm working on. Thanks for the help Zen.
1084
« on: January 14, 2018, 06:36:49 PM »
Despite this though, I doubt that anytime in future we will solely rely on AIs.
Also, real quick, the concern here is not that we will solely rely on AIs. The issue is that mathwashing (see the link I posted earlier) will affect the way we view and treat the output provided by intelligent computers. Experts aren't worried about an enormous AI controlling everything and directly making decisions without any human involvement. They're worried that people will blindly trust potentially flawed computers. This isn't about "Zen has applied for a job or a loan > AI analyzes the application > gives negative outcome potentially based on flawed data or analytics > AI rejects application and shelves it". It's about "AI analyzes the application > produces negative advice based on potentially flawed data or analytics > human in the loop (HR person, for example) blindly trusts the judgment of the AI and rejects the application". And there's a lot of reasons to assume that'll be the case, ranging from confidence (this is an enormously intelligent machine, why doubt it?) to accountability (if you reject the computer and something goes wrong, it's all on you and you're going to take the fall for thinking you're smarter than an AI) to complacency (people trust tech despite not knowing how it works). If you assign kids an online test or homework that is graded by the teaching platform, you're not going to manually check every single answer for every single student to see if the automatic grading system got it right. You're going to trust the system unless someone complains that their grade is wrong. If you're driving down a road and your car's dashboard says you're doing 70 (the speed limit), you're going to trust that the computer is correct and not assume you're only doing 60 and can still go 10 miles faster. And when you arrive at an intersection and the traffic light is red, you're going to trust the computer in the light and stop. When the first mainframe computers became a thing in the 60s and 70s, companies still had human "computers" (actual term used for these people) whose only job was to run the calculations of the machine and see if they were correct. Now, we put a lot of trust in these machines without thinking twice. Obviously, we have good reason to because they're generally secure, accurate and reliable, but that doesn't mean the same holds true for emerging tech like AI. This isn't about a dystopian AI solely deciding everything with humans being out of the loop entirely. It's about AIs spitting out analytics and advice that are effectively rubberstamped by people who don't even know how it works, who rarely are in a position to go against the AI and who blindly trust its accuracy.
1085
« on: January 14, 2018, 07:39:57 AM »
My guess is that the computer created generalizations that simplified the process of identifying animals in the quickest way possible. If I am correct, such a thing reminds me of the discussion of how AIs talking to one another "created" a new language by simplifying the syntax of English. That is indeed what AI's and machine learning systems are intended to do, but the reason I brought up this example is to show how this is prone to errors and misuse. There's dangers in using generalized profiles to make decisions about individual cases. Basically, the example is that, based on machine learning, this system was supposed to be able to differentiate between dogs and wolves. Dogs, especially some breeds like huskies and Malamutes, can look a whole lot like wolves, making this is a difficult task. As is always the case with these AI's, the system was trained by providing it with a lot of example pictures correctly marked "dog" or "wolf". By looking at the pictures and comparing them, the AI learns to pick up on the characteristics and subtle patterns that make dogs different from wolves. After it's done learning, the AI is then provided with new images so that it can apply the patterns it's learned and differentiate a dog from a wolf. As I already said, this worked well. It even worked so well that it raised suspicion. So what happened? As you correctly guessed, the system created generalizations of the pictures to make profiles of what a wolf looks like and what a dog looks like. Then, it matches new images to these profiles to see which one is the closest match. This was fully intentional, because the idea was that the system would look at things like color, facial features, body structure and so forth to determine which creature is which. However, and this is the kicker, the AI didn't look at the actual animals. Instead, it learned to look at their surroundings. The computer very quickly figured out that if the animal is surrounded by snow or forest, it's almost always a wolf. If it's surrounded by grass or human structures, it's almost always a dog. So rather than actually comparing the animals to one another, the AI basically whited out the shape of the dog or wolf and only focused on its surroundings to go "background = grass / stone so it's a dog" or "background = snow / forest so it's a wolf". This is a picture of a study that replicated this. Notice what the AI did? It blanks out the animal, looks at the surroundings, notices the snow and concludes it's a wolf. And in the vast majority of cases, it's entirely correct because it's a statistically proven fact that wolves are more likely to be found in dense foresty and snowy areas while dogs are found around human structures and grassy areas. But in several cases, this is also completely wrong. Wolves do venture out in grassy areas and sometimes can be found around human structures (take a picture of a wolf in a zoo, for example). Likewise, dogs do end up in the snow and foresty areas depending on where they live and where their owner takes them for walks. 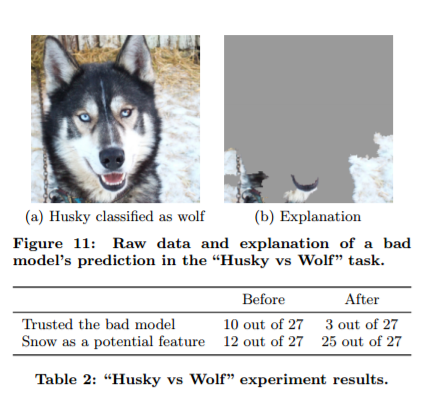 I brought this up to further illustrate my example from earlier. There are some serious disparate and negative effects that can come from, as you put it, "noticing trends" and applying them for decision-making without proper safeguards, oversight and mitigation techniques in place, even when they are based on solid, valid and statistically sound facts. And these things can and really do happen, partially because of how difficult it is to assess these systems and pick out flaws. Remember when Google's image recognition software identified black people as gorillas? After several years, we've finally found out what their "solution" is two days ago. Instead of fixing the acutal algorithm, which is a difficult thing to do even for a company like Google, they just removed gorillas from their labelling software altogether and made it into what's effectively an "advanced image recognition tool - for everything other than gorillas" package. And these things carry huge risks for everyone and in every field. Insurance, loans, job / college applications, renting or buying a place to live, courts, education, law enforcement... The more big data analytics are used (directly or indirectly) to make decisions about individuals or certain groups on the basis of general profiles and statistics, the larger the risk that we're going to see inequality grow and disparate treatments become even further institutionalized. In the eyes of the system, you're not just Zen based on your own merits, personality and past. Instead, you're a mix of 50% Zen and 50% "composite information of what the average Zen is expected to be like based on the actions of thousands of other people". If that latter 50% has a good connotation (white female from the suburbs in a good area with affluent college educated parents without a criminal past, for example), then that's great. But if it has a negative connotation (black male from the poor inner city and a single-parent household with poor credit score, for example), then Zen (no matter how kind, smart, motivated and capable) is going to systematically face more obstacles because the machine will be less likely to accept his job/college application, charge him more for insurance, refuse to grant him loans or rent him houses or appartments in certain areas, mark him as a more likely suspect for crime, subject him to more police surveillance and so on. And I think you're going to have to admit that this isn't fair, even when it's based on actual trends and stastics in our society, and that it can have serious consequences (the feedback loop of all these obstacles making him less likely to become successful which in turn reinforces the idea that people like him are less adequate and should be treated accordingly). Just to be clear though, I don't think we're really on opposite sides here. The point of this thread was just to get some Serious discussion going on an interesting topic. I don't oppose AI or these analytical systems being used by law enforcement at all. If I did, I wouldn't be working on cutting edge tech to be used by these people. This is just to raise awareness about the issues and bring up some of the potential issues and threats of things like mathwashing.
1086
« on: January 14, 2018, 06:50:14 AM »
I understand what you are saying about the feedback loops. However, to address that concern, this information should not be treated as the same data set, rather a subset of that data set. Like I discussed from my previous post, when an area is provided with an more intensive treatment for the purpose of remedying the difference between that area and the norm, the data should be used for analysis of how the area is improving over time. Think about it like a science experiment, the area is receiving a new variable into its equation (the increased police presence). Treating the area like the other areas is what supports that feedback loop. I feel like we're talking about two different things here as I don't see how your solution would address the problem. You seem to be talking about criminology studies where I agree that these datasets should be analyzed separately (which already happens). But that's only relevant for the perception of the area and evaluating the impact of new strategies over time. It doesn't really address the practical problems I've raised without crippling the system. Say you start using an analytical / predictive / big data / AI system for police and criminal justice authorities on January 1st 2018. It bases itself on all the data it has available to it from, say, 2000 - 2017. What would your solution entail over the following months? A police arrest on January 2nd goes into a separate dataset and is effectively kept out of the system? That would kind of defeat the purpose of having the system in the first place. Even at a policy level, I'm not sure how this would work. I agree with what you're saying as being important for criminological research and to assess the effectiveness of the data, but I don't really see how keeping a separate dataset would work to stop a feedback loop from occuring and from bad conclusions being drawn into the system.
1087
« on: January 13, 2018, 11:52:58 AM »
IRegardless, even with the systematic bias towards male teachers, I can say from my own personal anecdotes that metric has not as great of an effect as you think. I know it's not like that now, but my post relates to what can very well happen the more we rely on algorithms to make these decisions based on big data analytics. They learn patterns and apply them back in a way that ultimately reinforces them. Even if you explicitly tell a system not to look at whether someone is male or female, there's plenty of proxies it can use to indirectly tell someone's gender. You say it's fair to consider these things (which it very well might be), but the stronger the pattern the larger the "weight" assigned to the value. It's easy to say that it's fine for you right now and that you don't mind them considering this, but imagine a computer deciding a woman with lower qualifications and less experience than you should get the job because she is a woman (which the majority of teachers are, so it considers this "good" and the norm) and because you're a man (which ties to higher rates of sexual abuse, physical violence and overall a higher rate of getting fired). Would that still be fair? And this can and does go pretty far. You're applying for jobs or colleges. Your profile is checked and scored based on how well you would do. Aside from your own qualifications (degree, experience, traits), you're also scored against a general profile made of you based on similarities and the information they have on you. Your name sounds foreign or Spanish? Shame, but -10 points on language skills because statistically those people are less fluent in English than "Richard Smith" is. You're from area X? Ouch, well that place has some of the highest substance abuse rates in the country so you'll get a -10 on reliability because, statistically speaking, you're more likely to be a drunk or drug addict. You went to this high school? Oof, people from that school tend to have lower graduation rates than the national average so that's a -10 on adequacy. Your parents don't have college degrees? Sucks to be you but it's a fact that children of college educated parents are more likely to score well on university level tests, so -10 on efficiency. That's -40 points on your application based entirely on hard, solid and valid statistics or facts. Perfectly reasonable, no? Only, it aren't facts about you. It's facts about people like you taken on average. And of course, this will hold true for many like you. They won't do as well, they will fail more and they might in the end drop out. But for many, this doesn't ring true. They aren't drunks, they are motivated, they would get good grades and they do speak English well. But in the end, they don't even get the chance to try because the system rejects them. This likely condemns them to worse jobs, a lower education and ultimately an almost guaranteed lower social status, all while people from a "good" area with rich, white parents get more opportunities so that the inequality and the social divide grows while social mobility drops. Obviously, this is an exaggeration. It doesn't happen now, but it very well could in the not so distant future. As machine learning and AI become more commonplace and powerful, and the amounts of different data they are fed with continues to grow, it becomes increasingly difficult to ascertain exactly what goes on in their "brain". And as these systems are almost always proprietary and owned by companies, there's almost no real way to look into them and find out how they work - especially not if you're just an ordinary person. And in the area of policing and criminal justice, this is just as big of a problem. It's actually one that we're already seeing today. In several US states, there's a program being used called COMPAS. It's an analytical system that evaluates criminals to assess their risk and likelihood of recidivism. Based on information about them and a survey they're administered, it generates a score showing how likely this person is to commit crimes again. This score is used for several things, from judges to determine their sentence or the bail amount to parole officers deciding on their release. Sounds good, right? Well, there's two big problems. One, no one knows how it really works because the code is proprietary. Two, there's been studies done finding that it's racially biased. The general conclusion was that black defendants were often predicted to be at a higher risk of recidivism than they actually were, while whites were predicted to be lower risk than they actually were. Even when controlling for prior crimes, future recidivism, age, and gender, black defendants were 77 percent more likely to be assigned higher risk scores than white defendants. Is this because the system was built by a racist or was intentionally programmed to be racist? No, it's because of hard and factual data being used to treat people based on a profile. I'll probably finish the rest tomorrow in a shorter version.
1088
« on: January 12, 2018, 07:42:22 AM »
Sorry for the late reply by the way, I just got home recently, started writing, and got logged off by accident because I forgot to click the keep me logged on button.
No worries, take your time. I'll respond in parts to keep it digestible and make it so that I don't write it all at once. Got a bit carried away with this section though, so I'll keep it shorter for the next. I agree with everything you say in the first paragraph (how socioeconomic status affects crime rates and why they’re typically higher among minorities), but I think you’re kind of missing my point. No one is really “blaming” the data here, at least not in the way I think you’re interpreting it. When people say that big data is biased or dangerous, they don’t necessarily mean that it’s evil, wrong or intended to discriminate, but they are usually referring to one of two things. One, that the data itself is flawed because it’s based on inaccurate information (for example, a dataset of the race of possible suspects as identified by eye witnesses which is faulty because, as studies have shown, people are much more likely to fill in the gaps in their memory with prejudices so that white offenders are often mistakenly identified as blacks by victims) or because it’s collected in a skewed way (misleading sample – an analysis of how men deal with problems based entirely on information about prisoners convicted for violent crimes but representing men as a whole, for example). Two, that the analysis of potentially accurate data and decisions made on the basis thereof cause biased, adverse and harmful effects against a certain group of people or individuals. For example, poor people are more likely to commit (certain) crimes. You’ve already explained why (socioeconomic status leads to less opportunities and more likely involvement in crime). This is a fact that’s backed up by statistics and hard data. However, if you feed that kind of information to a learning algorithm (or it figures it out on its own), it can cause biased and dangerous results. - Information: most people involved in a certain type of crime are poor. - Correlation: poor people are a risk factor for committing crime. - Outcome: if two suspects are equal/identical in every way with the exception of their income, the poor person should be the one who is arrested and who should receive a harsher punishment, as he is more likely to have committed this crime and potential future crimes. - Outcome 2: the poor person is now arrested and receives a harsh sentence, which is information that goes back into the system. This reinforces the belief that poor people should be arrested more and receive harsher punishments because the algorithm was proven “right” last time (in the sense that the police and criminal justice system followed its lead – regardless of whether or not the person was actually guilty), meaning that the algorithm is even more likely to target poor people next time. All of this is based on solid data. It’s a valid and legitimate representation of an aspect of our society. Yet still, it can clearly cause disparate issues. If you let a big data analytical system tasked with finding patterns and learning about profiles into the decision-making process, you can definitely cause problems even though they seem based on solid information. Poor people are more involved in crime > more police focus on poor areas, less on richer areas > more poor people arrested and convicted > even more evidence that the poor have criminal propensity > even more focus on the poor (reinforcing the “prison pipeline” and putting more of them in prison, which we know teaches criminal habits and make them less likely to be employed afterwards so they remain poor) and even more sentences and harsh judgments > system grows increasingly more “biased” against poor people because of the feedback loop (it’s being proven “right” because more poor people are going to jail because of it) > the current problems of inequality persist, the underlying problem goes unaddressed, minority communities are further ostracized, the rich/privileged are given more “passes” while the poor/disadvantaged are given less leeway and more punishments > social mobility is stifled and the divide between the rich and poor grows because institutionalized computer systems serve as an added obstacle… And all of this happens on the basis of cold, hard and factual data paired with a very smart computer. This is just one of the dozens of possible scenarios, but I hope that this clarifies what I meant. Data is not necessarily wrong or inherently bad, even when it’s “biased”. The point is that technology can pick up on these inequalities / problems / different treatments and actually reinforce them further because it considers them the norm. The risk is that algorithms learn from data, create generalized (and potentially “prejudiced” or “biased) profiles, and then apply them to individuals (“your father was abusive which means that you’re more likely to be abusive too so you’ll be denied a job since you’re considered a potential abuser regardless of the person you are”) which suffer as a consequence but have almost no way to fight back because their disparate treatment is (often wrongfully) legitimized as “well the computer says so and it’s an intelligent piece of technology so it’s neutral and always objective”. That said though, I'm not at all against these new technologies. I think they carry great promise and can be very useful. I even work on them to provide legal advice and aid their development in legally and ethically compliant ways. I just wanted to point out some of the dangers and hear what people thought of it.
1089
« on: January 11, 2018, 11:32:50 AM »
I got stuck on a bullshit 4 day trial where some dipshit put a pole through a bar's window and ran from the police for 6 blocks. His fingerprints and blood were on the pole and had several cops literally watching him for his run. He said someone else did it.
Yeah, we said guilty in like 5 seconds
I've always been surprised by the kind of cases the US and UK require a jury for.
Every criminal trial, bro. It's in the constitution.
Yeah, I know. Always seemed a bit excessive to me.
Rights we consider to be fundamental seeming frivolous to Flee?
I want every major news outlet on the phone immediately, they're going to shit themselves when they hear about this development.
Well that's a pretty spirited reaction over a very mild criticism of an aspect of the US justice system.
1090
« on: January 11, 2018, 11:26:47 AM »
What you're saying is a common misconception. You're missing a few key things, which I'll try to explain briefly. 1. Statistics are easily manipulated and interpreted in different ways. They're a guideline but insufficient to dictate policy on their own and prone to misuse. The real problems arise when big data is used not just to provide information but to make decisions affecting individual people. Imagine you're a man looking to become a teacher. The school district employs an algorithm to assess job applications and potential candidates. This system takes into account dozens of characteristics and data points to evaluate your profile. One of the things it learns from the data it's trained with is that men make up the vast majority of sex offenders and are responsible for almost all cases of teachers sexually or physically abusing students. As a result, it ties men to these crimes and incorporates this into its decisionmaking. Every man, by default, gets a point deduction because they're a higher risk profile and will systematically be hired less. This goes for a dozen different things. Say you're applying for a college. Its algorithm determines that people from your state / area / region / background tend to drop out more often than the average. Since every student is an investment, colleges want successful ones. As such, your name is by default put at the bottom of the list despite no person at the school having met you or being able to assess you on your merits alone. The same thing applies here. All of this, as you say, is based on accurate, real and reliable facts that notice trends in our society, yet I think you're going to have to agree that it's far from fair. 2. These algorithms exacerbate existing problems and biases by creating a feedback loop. Say the system identifies an area or a specific group that has a lot of issues with crime. As a result, the police focuses its attention there and deploys more cops with a specific mandate. You will see that even more crime is now recorded in this area, merely due to the fact that there's simply more cops that are actively looking for it. There's not any more crime that there was before, but it's just noticed more often. You then put this new data in the system and voila - feedback loop. "The computer was correct, we listened to it and caught more criminals who are black". This can lead to adverse effects and the over / under policing of certain areas. The attributes you've found serve as a proxy for race and rather than fairly policing anything, you're now effectively policing people based on the color of their skin. Take the policies like stop and frisk or random traffic stops. There's been a lot of research into this finding substantial racial bias in how they were executed. If you now use that date to train a computer to determine who should be "randomly" stopped, you'll find that it also focuses more on blacks. Aside from the problem of how this affects innocent individuals (see 1.), simply by focusing more on blacks, you'll now find more criminals among them. That's basic logic. Feed this back into the system and you'll end up with a situation where whites are given a pass or stopped less and less based on the assumption that they're less likely to be criminals, but this assumption is already based on previous data (analysis) and can therefore exacerbate the issues and bias. This can lead to the underlynig problem being ignored and existing problems being continued rather than fixed. 3. You now also institutionalize the problem. It's easy to check a person and have them justify certain actions in order to determine if they're prejudiced or wrong, but it's a lot harder with a very intelligent computer. People take what technology says for granted and trust that it's neutral, fair and accurate, while it very often isn't. The more we move towards machine learning, the more we run the risk of incorporating these issues that are potentially extremely difficult to detect. A famous example is that of image recognition software distinguishing between different animals. An AI was trained to do this and it became extremely good at it with very little effort. So good that the people who created it became skeptical. Want to take a guess what they found when they really put it to the test? I'll comment later.
1091
« on: January 11, 2018, 09:58:51 AM »
I even skimmed through the Nintendo Direct to see this announcement.
1092
« on: January 11, 2018, 09:48:06 AM »
I hope he wins not even for political reasons but because fuck Google.
Why fuck Google?
1093
« on: January 10, 2018, 07:26:50 PM »
Quake 3 / Live: low player count due to how difficult it is to get into and the lack of good tutorials is the only flaw I can really think of. Everything else is sublime.
Thoughts on snowball effect
In Quake duel? It's a possibility, but not necessarily detrimental. The playing in / out of control aspect is vital. The only way a snowball would occur in a match between similarly skilled players is if one of them would play absolutely perfectly and the other failed. One, keeping perfect control is extremely hard to do. Two, there should pretty much always be opportunities for the player out of control to get himself back into the game.
1094
« on: January 10, 2018, 05:57:38 PM »
Quake 3 / Live: low player count due to how difficult it is to get into and the lack of good tutorials is the only flaw I can really think of. Everything else is sublime.
1095
« on: January 10, 2018, 05:54:24 PM »
How does one get summoned for it? Maybe it isn't a thing in the middle of nowhere (Wales)
Should still be a thing. You basically get a letter in the mail saying that you've been randomly selected based on the court and where you live. If you meet the eligibility requirements and don't file a good reason to abstain, you're expected to show up at the pre-trial where each party gets to dismiss possible jurors.
1096
« on: January 10, 2018, 04:04:41 PM »
I got stuck on a bullshit 4 day trial where some dipshit put a pole through a bar's window and ran from the police for 6 blocks. His fingerprints and blood were on the pole and had several cops literally watching him for his run. He said someone else did it.
Yeah, we said guilty in like 5 seconds
I've always been surprised by the kind of cases the US and UK require a jury for.
Every criminal trial, bro. It's in the constitution.
Yeah, I know. Always seemed a bit excessive to me.
1097
« on: January 10, 2018, 01:03:04 PM »
Video is very short, but the tl;dr is that technology isn't neutral and machines can and do learn bad things from us. Among others, this is particularly troublesome in the area of law enforcement and criminal justice. The rise of big data policing rests in part on the belief that data-based decisions can be more objective, fair, and accurate than traditional policing.
Data is data and thus, the thinking goes, not subject to the same subjective errors as human decision making. But in truth, algorithms encode both error and bias. As David Vladeck, the former director of the Bureau of Consumer Protection at the Federal Trade Commission (who was, thus, in charge of much of the law surrounding big data consumer protection), once warned, "Algorithms may also be imperfect decisional tools. Algorithms themselves are designed by humans, leaving open the possibility that unrecognized human bias may taint the process. And algorithms are no better than the data they process, and we know that much of that data may be unreliable, outdated, or reflect bias."
Algorithmic technologies that aid law enforcement in targeting crime must compete with a host of very human questions. What data goes into the computer model? After all, the inputs determine the outputs. How much data must go into the model? The choice of sample size can alter the outcome. How do you account for cultural differences? Sometimes algorithms try to smooth out the anomalies in the data—anomalies that can correspond with minority populations. How do you address the complexity in the data or the "noise" that results from imperfect results?
Sometimes, the machines get it wrong because of racial or gender bias built into the model. For policing, this is a serious concern. [...]
As Frank Pasquale has written in his acclaimed book The Black Box Society, "Algorithms are not immune from the fundamental problem of discrimination, in which negative and baseless assumptions congeal into prejudice. . . . And they must often use data laced with all-too-human prejudice."
Inputs go in and generalizations come out, so that if historical crime data shows that robberies happen at banks more often than at nursery schools, the algorithm will correlate banks with robberies, without any need to understand that banks hold lots of cash and nursery schools do not. "Why" does not matter to the math. The correlation is the key. Of course, algorithms can replicate past biases, so that if an algorithm is built around biased data, analysts will get a biased result. For example, if police primarily arrest people of color from minority neighborhoods for marijuana, even though people of all races and all neighborhoods use marijuana at equal rates, the algorithm will correlate race with marijuana use.
The algorithm will also correlate marijuana with certain locations. A policing strategy based on such an algorithm will correlate race and drugs, even though the correlation does not accurately reflect the actual underlying criminal activity across society. And even if race were completely stripped out of the model, the correlation with communities of color might still remain because of the location. A proxy for racial bias can be baked into the system, even without any formal focus on race as a variable. [...]
As mathematician Jeremy Kun has written, "It’s true that an algorithm itself is quantitative—it boils down to a sequence of arithmetic steps for solving a problem. The danger is that these algorithms, which are trained on data produced by people, may reflect the biases in that data, perpetuating structural racism and negative biases about minority groups."
Big data policing involves a similar danger of perpetrating structural racism and negative biases about minority groups. "How" we target impacts "whom" we target, and underlying existing racial biases means that data-driven policing may well reflect those biases. This is a much bigger problem than most people realize. It's only really entered the spotlight over the past two or three years and is only just now becoming mainstream. Figured I'd make a thread about it to bring some life to Serious and because this is what I am currently working on (making AI accountable).
1098
« on: January 10, 2018, 12:45:13 PM »
I got stuck on a bullshit 4 day trial where some dipshit put a pole through a bar's window and ran from the police for 6 blocks. His fingerprints and blood were on the pole and had several cops literally watching him for his run. He said someone else did it.
Yeah, we said guilty in like 5 seconds
I've always been surprised by the kind of cases the US and UK require a jury for.
1099
« on: January 10, 2018, 12:41:20 PM »
You see, I was eagerly awaiting to get a summons in the mail for my first time. It wasn't until I finally went to jury duty when I realized it was fucking awful. Next time I'm taking my copy of Mein Kampf and hope they mark me down to never summon again.
Although I now kinda wish I got to do it at least once. Yeah, I realize it's not very interesting. Only reason I'd want to do it is because of my legal background, really. And ironically enough, that's also exactly what's made it so that I can't be summoned.
1100
« on: January 09, 2018, 07:57:57 PM »
>tfw I'm exempt from jury duty forever
Feels good.
Although I now kinda wish I got to do it at least once.
1101
« on: January 09, 2018, 05:17:09 PM »
The Guardian - James Damore sues Google, alleging intolerance of white male conservatives Class-action lawsuit led by fired engineer includes 100 pages of internal documents and claims conservatives are ‘ostracized, belittled, and punished’
Google is facing renewed controversy over its alleged intolerance toward conservatives at the company, after a class action lawsuit filed by former engineer James Damore disclosed almost 100 pages of screen shots of internal communications in which employees discuss sensitive political issues.
The evidence appended to the lawsuit, which was filed on Monday, includes a message from Rachel Whetstone, who worked as a senior Google executive after a career in the UK Conservative party, bemoaning “prejudiced and antagonistic” political discourse at the company.
Damore, who was fired in 2017 after writing a controversial memo about gender and technology, alleges in the lawsuit that white, male conservative employees at Google are “ostracized, belittled, and punished”.
The lawsuit claims that numerous Google managers maintained “blacklists” of conservative employees with whom they refused to work; that Google has a list of conservatives who are banned from visiting the campus; and that Google’s firings of Damore and the other named plaintiff, David Gudeman, were discriminatory.
“We look forward to defending against Mr Damore’s lawsuit in court,” a Google spokesperson said in a statement.
The company’s workforce, like much of the rest of the tech industry, is overwhelmingly white, Asian, and male. In 2017, the US Department of Labor accused Google of “extreme pay discrimination” against women, and a group of women have filed a class-action lawsuit against the company alleging systemic wage discrimination.
But the Damore lawsuit purports to expose a cultural bias toward promoting diversity and “social justice” that, the suit claims, has created a “protected, distorted bubble of groupthink”. Efforts to increase the representation of women and underrepresented racial minorities, which companies like Google have undertaken in response to external criticism, are cast in the suit as illegal discrimination against the majority.
Screenshots of internal communications reveal numerous employees appearing to support the idea of being intolerant toward certain points of view, such as one post arguing that Google should respond to Damore’s memo by “disciplining or terminating those who have expressed support”. In another post, a manager stated his intention to “silence” certain “violently offensive” perspectives, writing: “There are certain ‘alternative views, including different political views’ which I do not want people to feel safe to share here … You can believe that women or minorities are unqualified all you like … but if you say it out loud, then you deserve what’s coming to you”.
Internal posts discussing the debate around diversity at Google, such as a meme of a penguin with the text “If you want to increase diversity at Google fire all the bigoted white men”, are filed as an appendix to the lawsuit under the heading “Anti-Caucasian postings”.
One manager is quoted as posting: “I keep a written blacklist of people whom I will never allow on or near my team, based on how they view and treat their coworkers. That blacklist got a little longer today.” Another screenshot reveals a manager proposing the creation of a list of “people who make diversity difficult”, and weighing the possibility that individuals could have “something resembling a trial” before being included.
In the 2014 email from Whetstone, who served as senior vice-president of communications and public policy at Google for several years before departing for Uber, she wrote: “It seems like we believe in free expression except when people disagree with the majority view … I have lost count of the times at Google, for example, people tell me privately that they cannot admit their voting choice if they are Republican because they fear how other Googlers react.”
The complaint argues that Google’s tolerance for “alternative lifestyles” – the company has internal mailing lists for people interested in “furries, polygamy, transgenderism, and plurality” – does not extend to conservatism. One employee who emailed a list seeking parenting advice related to imparting a child with “traditional gender roles and patriarchy from a very young age” was allegedly chastised by human resources.
The suit also alleges that Google maintains a “secret” blacklist of conservative authors who are banned from being on campus. Curtis Yarvin, a “neoreactionary” who blogs under the name Mencius Moldbug, was allegedly removed from the campus by security after being invited to lunch. The plaintiffs subsequently learned, it is claimed in the suit, that Alex Jones, the InfoWars conspiracy theorist, and Theodore Beale, an “alt-right” blogger known as VoxDay, were also banned from the campus.
The suit will likely reignite the culture wars that have swirled around the tech industry since the election of Donald Trump. Many liberals within the tech industry have pressured their employers to take a stand against Trump policies, such as the Muslim travel ban, and companies have struggled to decide the extent to which they will allow the resurgent movement of white nationalists to use their platforms to organize.
Damore’s firing in August last year was heavily covered by the rightwing media, which portrayed the saga as evidence of Silicon Valley’s liberal bias, and the engineer was transformed into a political martyr by prominent members of the “alt-right”.
In making its case against Google, the suit reveals some of the internal backlash Damore received after his memo went viral, including a mass email in which a Google director called the memo “repulsive and intellectually dishonest” and an email to Damore from a fellow engineer stating: “I will keep hounding you until one of us is fired.”
Gudeman, the second named plaintiff, was fired following a post-election controversy in another online forum at Google. A Google employee posted that he was concerned for his safety under a Trump administration because he had already been “targeted by the FBI (including at work) for being a Muslim”. According to the suit, Gudeman responded skeptically to the comment, raising questions about the FBI’s motives for investigating the employee, and was reported to HR.
Gudeman was fired shortly thereafter, the suit claims, after Google HR told him that he had “accused [the Muslim employee] of terrorism”. The public reaction to this is of course very reasonable, nuanced and well thought out.
1102
« on: January 09, 2018, 08:48:00 AM »
I'm just going to repeat the last sentence of my post and call quits because we're clearly not on the same page here. "You can realize something is for the better and still wish it wasn't so."
1103
« on: January 08, 2018, 04:06:56 PM »
So what is the difference between the animation here? I haven't really noticed any big drops in quality or whatever.
1104
« on: January 08, 2018, 04:06:01 PM »
You're kind of missing the whole "I wasn't really being serious" part. This was about whether you or I want there to be a hiatus in the show. I don't, because I'm enjoying it and just want it to keep going at the same rate and in the same quality. It's like saying "fuck that" to the next Star Wars movie not coming out for another 2 years, even though you well know that there's no actual way they can release it today as shooting hasn't even started yet. You can realize something is for the better and still wish it wasn't so.
1105
« on: January 08, 2018, 02:17:46 PM »
Lets hope the rumours of a hiatus after this arc are true
Fuck that.
The show desperately needs to catch a break. There's no good reason to not have one and all its going to do is make both the movie and the show worse.
"I don't want to wait" is a perfectly good reason though.
Spoiler Not actually being super serial here
That's just dumb though. Who cares if there's a new episode every week if nothing interesting happens and they look bad? Imagine if from the beginning of March to the end of December all we got were filler episodes. No one can die, no one can get a new form, no one can really change or grow. On top of that, it will all look sub par because the animators will be forced to work on the show in between moments of working on the movie. It's not a good idea.
It's only dumb if you accept the limitations. If LC's correct, the people making the show are raking in cash. There is no reason episodes couldn't be ongoing (bi-weekly, for example) and made well in advance provided that they expanded the team and whatnot. This isn't an either X or Y kind of deal. Of course I want quality over quantity, but that doesn't mean you can't want both and wish there wouldn't be a long hiatus when there is a theoretical alternative.
1106
« on: January 08, 2018, 11:18:33 AM »
Lets hope the rumours of a hiatus after this arc are true
Fuck that.
The show desperately needs to catch a break. There's no good reason to not have one and all its going to do is make both the movie and the show worse.
"I don't want to wait" is a perfectly good reason though. Spoiler Not actually being super serial here
1107
« on: January 08, 2018, 10:53:52 AM »
Lets hope the rumours of a hiatus after this arc are true
Fuck that.
1108
« on: January 08, 2018, 06:07:55 AM »
time was your ally alphy, but now it has abandoned you
This sep7agon forum... is now yours
1109
« on: January 08, 2018, 02:23:26 AM »
When I watched Rick and Morty with my friends and I was the only one to understand the subtle philosophical references and quantum physics in the show. Felt like I've been surrounded by simpletons ever since.
1110
« on: January 07, 2018, 07:05:17 AM »
Good episode.
Pages: 1 ... 353637 3839 ... 520
|